Data-Centric AI with Snorkel and MinIO
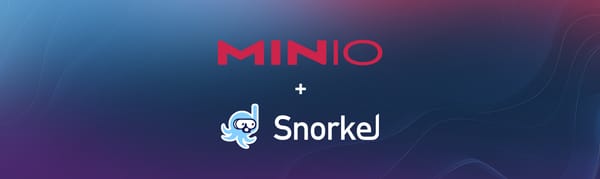
With all the talk in the industry today regarding large language models with their encoders, decoders, multi-headed attention layers, and billions (soon trillions) of parameters, it is tempting to believe that good AI is the result of model design only. Unfortunately, this is not the case. Good AI requires more than a well-designed model. It also requires properly constructed training
Read more...